Scaling AI in Retail - How can Brick-and-Mortar Retailers Leverage AI for Business Growth?
Scaling AI in retail can transform the retail landscape for customers as well as sellers by driving customer engagement and giving actionable insights to retailers. While eCommerce companies have been leveraging AI in new ways, physical retail stores are slower in adopting AI in their processes. In this article, we discuss-
- Why are eCommerce companies better placed to leverage AI than retail stores?
- Challenges for retailers in adopting AI.
- How can retailers solve those challenges and leverage AI the same way as eCommerce.
A very interesting paradox has developed over the last two decades, where two very similar markets serving the same need have fared very differently in their success using technology. The technology being AI and the two markets being traditional retail stores and eCommerce. Giants in eCommerce such as Amazon, Alibaba, Flipkart and others, were set up perfectly from their very early days to be successful in their AI journey owing to their entirely digital nature in interacting with the customers. This helped them not only monitor what their customers were doing but also in trying to understand what their potential customers were not doing.
One of the very early data analysis use cases in ecommerce dating back to the early 2000s, was the prevailing concern about abandoned shopping carts in online stores. Ecommerce retailers wanted to understand and do everything possible to motivate their customers in completing their transactions and often following them to their inboxes to encourage or in many cases irritate them to go back and buy the stuff that they may just have been window shopping. In fact, those processes continue even to this day, extending beyond ecommerce stores into other online commerce activities and happen more aggressively with you being reminded of your intention to buy something somewhere, whatever device you happen to be on whether it is a laptop, phone, tablet or your watch etc.
Despite the stalking nature of those methods, they seem to be working with digital commerce becoming more mainstream than it was several years ago. People seem more comfortable spending their money online for goods they probably have not seen in person. The success in this space can be owed entirely to how digital commerce leveraged the data it collected from its users.
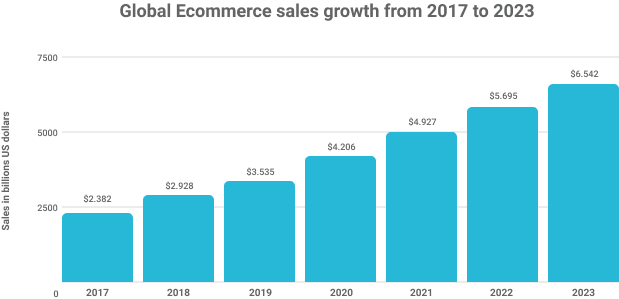
While initial efforts to track and understand user behavior tended to be more invasive and nefarious, the ecommerce industry has adapted to more intelligent techniques to track and understand its user/prospect/buyer behavior to start catering to their needs, personalizing their experiences and anticipating their demand very effectively. AI has played a significant role in all of these aspects and some more with improving safety for online shoppers, reducing the amount of fraud and also protecting their purchases.
We could dedicate an entire article to AI in ecommerce but we are here to talk about AI in retail. One clear advantage that eCommerce has over traditional retail is the access to data. This doesn't necessarily only mean a buyer’s personal data but when coupled with ancillary information such as general trends in online shopping behaviors by demographics, seasons, social media trends, weather patterns and geopolitical events; Digital traders have access to intelligence more readily and in better correlations to real-time data compared to their real life counterparts.
Scaling AI in Retail – The ‘What’ and the ‘How’
So what can be done such that AI can help Retail the same way it helped Online commerce. The “what” in this case can be identified relatively easily which is analyzing data to identify buyer intent, anticipating product demand better in the short term than long term, reacting to changing customer preferences in a near real-time fashion and focusing on personalization of customer experiences when shopping in person. If that establishes “what” needs to be done, the actual implementation of the processes are the “how” and that's where the challenges begin.
Let's look at those a bit more closely and explore how we can simplify the hurdles.
Customer Intent and Short-term Product Demand
This is where a world of difference exists between ecommerce and traditional retail. Digital traders have easy access to customer data to understand their intent and at the same time have a relatively lower threshold to overcome in stocking goods by using a central warehouse to cater to users spread over large demographic areas. They also enjoy the luxury of time in delivering those goods. Retailers on the other hand have to be hyper focused on what buyers in their immediate geographic vicinity might be interested in buying and be prepared to serve those products readily when the shopper walks in the door.
Retailers in this case can depend on the same data as their digital counterparts to understand buyer intent but will need to narrow down their focus on data specific to their geographic location. Challenges in accessing and understanding customer intent is not straightforward and requires processing billions of data points to achieve a high degree of accuracy to identify consumer intent at a regional, category and an item level.
Luckily, this data is becoming increasingly accessible with specialists that focus on capturing such data points and transforming them into insights helpful for retailers. Centricity Insights is one such company that is making rapid advancements in capturing this data and transforming it into real-time actionable insights for category managers in retail stores.
Adapting to Customer Preferences
Ecommerce stores have a clear advantage here, anytime a user searches for a product on their store, reads a review, posts a question or completes a transaction, it’s one additional data point that a digital store can capture from its users. This can be anonymised as well without revealing user identity but very effectively capturing user interests and preferences within their stores. The retail store equivalent of this would be tracking a user's every move, aisle browsing patterns and frequently bought products in a real life setting where the data is harder to translate into measurable data points.
Advances are being made in this space as well with IoT sensors that can capture a variety of data points such as proximity, pressure, movement and images. With proper segregation of products and an extensive distribution of such sensor inputs, a wide variety of data points can be captured such product categories that attract more eyeballs, the correlation between aisle traffic and transactions at a category and product level, aisle congestion allowing for better distribution of products and also being able to evaluate shelf allocations for products based on demand.
All of the above in conjunction with the physical transaction data provide for a rich dataset that can be translated into real-time actionable insights about buyer preferences and behavior in an extremely localized scenario.
Personalization
How can an individual shopping experience be improved? This is where digital and retail entities should join forces to make any individual shopper’s experience better as soon as one steps into a store. In my personal shopping behavior, I tend to frequent the same store most often and typically my buying patterns can be easily predicted if anyone is capturing my actions as data points. Stores already have an easy way of doing this with loyalty cards. If the same store has a digital presence in the form of a website or an app, it would make sense to correlate my buying patterns with online trends and start with simple recommendations before moving into more personalized recommendations.
The challenges here may not just be technical but more social in nature. People in general value their privacy and don’t forgive intrusion easily. Being reminded by an app in the middle of a day to go and buy something you may need but not that very instant can be considered intrusive. So location based targeting when a user is physically near a store or inside the store might be more effective and would actually be helpful. More points, if the recommendation actually meets the needs of the buyer. While the logistics of the buyer presence is a software challenge, identifying and recommending the right products to a customer is where AI can be effective. This is the real life equivalent of “You may also like this…”, that we so often see in online stores.
My intention is to not say that Retail has not taken advantage of AI, it is more to identify the clear advantage online commerce has in reacting to buyer behaviors and trends more quickly.
The opportunities and challenges detailed above do not discount the general challenges organizations face with scaling AI effectively. However, addressing all these hurdles is becoming increasingly manageable as we see advances in AI and intelligent software development processes in general.
For smaller retail organizations where budgets for AI initiatives may be constrained, the same results compared to larger entities can be achieved by entering into a partner ecosystem with complementary skill sets. There are several open-source AI solutions that can be leveraged by smaller organizations to get a running start, low-cost Big Data frameworks that can be set up using AWS and Azure cloud environments and extensive libraries of existing AI use cases in Github repositories that have already solved some impressive problem statements. In addition, there are organizations that offer Data Science, Data Management, and AI as services that can be subscribed by organizations that do not have the capability to successfully manage these in-house or dedicate resources to implement them successfully.
At Qualetics, we specialize in playing the role of the Intelligence Strategy partner by providing guidance to our clients in identifying the best use cases for their data and seeing through the implementation with our proprietary AI platform. Reach out to us should you see your organization benefiting from opportunities in implementing AI.