Education in the time of Covid-19, How can AI help?
Covid-19 is causing K-12 and post-secondary education to replace traditional classrooms with the relative safety of virtual learning. Most schools were unprepared for such a sudden shift in their practices leading to tremendous innovation from educators as they serve the needs of students and their families and persevere through each day’s new challenges. And for students and their families the changes have been difficult as they adjust to connecting virtually with teachers and classmates turning homes into learning environments while many parents are adjusting to sharing the same space to work.
“Forward movement is not helpful if what is needed is a change of direction.”
― Dr. David Fleming, Lean Logic: A Dictionary for the Future and How to Survive It
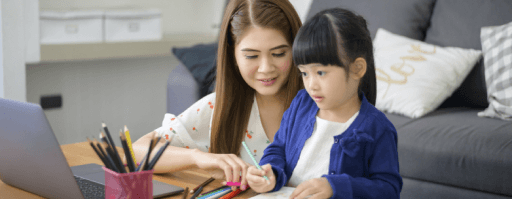
Because these changes have occurred so quickly the public is concerned about how effective these educational changes will prove to be. Current research shows parents of K-12 students and adults considering enrolling in post-secondary college or vocational training share similar concerns about value and access to education. (Respective references are Pew Research Center,How Parents of K-12 Students Learning Online Worry About them Falling Behind, October 2020, and Strada, Center for Consumer Insights, Back to School: Perspectives from the American Public, August 2020)
So in the midst of this disruption we have the opportunity to embrace the changes and develop innovations that deliver new levels of learning effectiveness. Here are a few ways AI can enhance virtual learning effectiveness some of which may relate more to K-12 than to post-secondary environments and vice-versa:
Personalized Learning: The millions of people who use services like Amazon and Spotify have firsthand experience with personally curated choices. Millions if not billions have been invested in personalization algorithms with commercial objectives. Similar algorithms adapted for education could have an even greater benefit to society to deliver personalized learning experiences.
Adaptive Learning: If personalized learning’s objective is to present the individual with a learning path based on their learning history, behavior, and preferences then adaptive learning’s objective is to optimize how much time and energy it takes to move through that path while mastering the learning goal. Adaptive learning detects whether the learner is struggling with a topic and presents more material on the topic in complementary ways helping the learner understand it better. This is similar to a teacher detecting one explanation or story not reaching the student so they continue the explanation with a similar but different explanation. And if the learner has already shown mastery of the topic the path is adjusted so it isn’t repeated unnecessarily though it may be reinforced in related topics. The result is a learning path optimized to achieve a learning goal in the most effective and least redundant manner.
Improve Confidence as well as Competence: Especially where children are concerned it’s important to develop their confidence as well as their knowledge level. Just as a system can score an answer right or wrong to address competence gaps the system can look for confidence indicators to deliver confidence-reinforcing cues, exercises, or assignments helping the learner become more aware of their mastery and more confident in themselves and their knowledge.
Referential Learning or Just-In-Time Learning Support: With the vast and growing universe of learning resources available, students rely on typical online search and lookup methods to find helpful information or guidance. Most of these searches are keyword based where results are returned based on an exact match to the search words. This requires the student to know what they are looking for, defeating the purpose of referential learning.
Just-In-Time learning support should be enhanced with the help of natural language processing (NLP) to analyze a student's free form text query such as “what are the differences between an epidemic and a pandemic?" and return references that deal directly with classifying diseases. An extension of this would be results that take into account the student's learning history and profile. If the student has been focused on airborne diseases in his own region, results could highlight content that might be regional.
This technology is prevalent in other forms such as modern search engines but is yet to make an impact in learning platforms.
Activity and Performance Metrics: Most people are familiar with traditional enrollment and completion reports presented in their tabular form. Today's analytics and data visualization capabilities can greatly advance our understanding of how learning activity and behaviors are connected to results. Instructional designers may find it very helpful to understand how students have navigated courses and which referential supplements were most helpful to students to optimize the course experience. And technical staff can monitor learner activity to plan maintenance periods. Having detailed information easily available visually presented in interactive form allows for more qualitative adjustments for your learners.
Predictive Analytics: Predictive algorithms can be developed to track a student’s learning behavior and competence levels to proactively engage the student if they are falling outside desired patterns. This can be particularly helpful in engaging the student or their parents about progress, trends, and predicted outcomes sooner allowing more time to adapt behaviors before students fall too far behind. And for more mature learners predictive indicators can make it easier for them to self-correct.
AI-enabled Intelligent Assistance: In a classroom the teacher has visual clues as to whether the student is attending to a topic or if they are listening but appear stuck. It can be as simple as the teacher asking the student if they need some help to engage in a dialogue that clarifies what may be a small but important point. Learner behavioral cues can be identified allowing an AI-enabled chat to engage a learner with a structured dialogue that results in specific guidance and knowledge being delivered or escalates the situation for personal attention having already captured helpful information.
AI and analytics can play a valuable role that enhances the learning experience and improves results and it’s likely easier to accomplish than you think. If you’re involved in education or edtech and would like to learn more about how any of these ideas could be applied please don’t hesitate to reach out, my associates and I here at Qualetics Data Machines want to help!